— Ella Wheeler Wilcox
“A man dies… only a few circles in the water prove that he was ever there. And even they quickly disappear. And when they’re gone, he’s forgotten, without a trace, as if he’d never even existed. And that’s all.”
– Wolfgang Borchert
“A man who wants to lead the orchestra must turn his back on the crowd.”
– Max Lucado, Christian author and preacher
Beyond Beyonce
- Philosophy and magic — an interesting interview with Jason Leddington (Bucknell) about his research and teaching on the subjects and how he got into it
Something in me
despite everything
can’t believe my luck
A poem isn't a puzzle to be solved but an experience to share
Data craft: the manipulation of social media metadata
New anti-terror laws to allow the bugging of a criminal's jail cell
The laws would allow the prison cells of any criminal suspected of posing a terror-threat to be put under surveillance
The dramatic moment that saw a political rumour turn into a front ...
The dramatic moment that saw a political rumour turn into a front ...
The rich really are different, and not just because they don't cut coupons. It often seems that they escape the rules that apply to the rest of us. Thus, there is understandable fascination when rich bad actors get a comeuppance. That is probably why so many folks blogged last week's decision about Wesley Snipes, where the Tax Court found that the Office of Appeals did not abuse its discretion in rejecting Snipes' OIC that would pay less than 4% of his $23.5 million tax liability. "Tax Girl" Kelly Erb put up this terrific post if you want the salacious details.
Lesson From The Tax Court: Last Known Address Rules Apply To The Rich And Famous Too
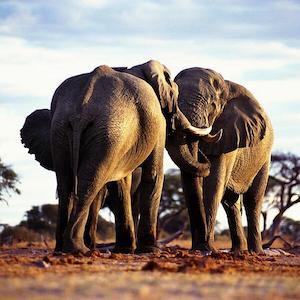
Book of the Day: Walkaway by Cory Doctorow P2P Foundation
Why
on-the-job training is failing to prepare public service leaders
CAPABILITY: Leadership development programs should call on senior managers to “get out from behind the email” and take a more active role in mentoring staff, says a study on the influence of the 70:20:10 learning model.
Labor pledges new evaluator-general as program experts’ collaborator
EVALUATION OFFICE: Rather than another adversarial watchdog sitting outside executive government, Labor’s proposed evaluator-general would sit inside Treasury functioning as a collaborative partner.
◾ Why Australia needs an evaluator-general
Before replacing a carer with a robot, we need to assess pros and cons
HEALTH CARE: It's easy to get excited about the potential for robots to help care for the sick, injured and elderly, but we need the right regulations in place to deal with issues as they emerge.
Open learning: the whole BX2018 nudge conference is out now on video
New ways of thinking about how people move around our cities
URBAN PLANNING: As population increases and cities become denser, how will the government usher in a new era of transport with mobility as a service? (Partner article
CAPABILITY: Leadership development programs should call on senior managers to “get out from behind the email” and take a more active role in mentoring staff, says a study on the influence of the 70:20:10 learning model.
Labor pledges new evaluator-general as program experts’ collaborator
EVALUATION OFFICE: Rather than another adversarial watchdog sitting outside executive government, Labor’s proposed evaluator-general would sit inside Treasury functioning as a collaborative partner.
◾ Why Australia needs an evaluator-general
Before replacing a carer with a robot, we need to assess pros and cons
HEALTH CARE: It's easy to get excited about the potential for robots to help care for the sick, injured and elderly, but we need the right regulations in place to deal with issues as they emerge.
Open learning: the whole BX2018 nudge conference is out now on video
New ways of thinking about how people move around our cities
URBAN PLANNING: As population increases and cities become denser, how will the government usher in a new era of transport with mobility as a service? (Partner article
What Empire Loyalists Are Really Saying When They Bash Julian Assange Caitlin Johnstone
5 recycling myths busted. National Geographic (J T McPhee). Panders to the mag’s middle class audience in touting recycling- although I won’t deny there will be some benefits to that. Overall, the piece is panglossian in its failure to confront the scope of necessary changes to prevent drowning ourselves in plastic
How The ‘House Of Cards’ Crew Rewrote The Entire Last Season Without Kevin Spacey
“[Spacey’s scandal] was a ‘gut punch,’ [co-showrunner Frank] Pugliese said, but the prospect of tossing out five months of work and having to rebuild the season without the show’s corrupt central figure actually emboldened him and his partner. ‘It felt so unfair to the story, in a way, we had to defend the world of the show,’ [co-showrunner Melissa James] Gibson said.” Here’s how they pulled it off.
Slate – Facebook and others have gotten more serious about hoaxes, hate speech, propaganda, and foreign election interference. Here’s how it helped in the midterms—and why they aren’t going away.
“At first grimace, the role of social media in the 2018 U.S. midterm elections looked a lot like the role it played in the 2016, when the hijacking of tech platforms by foreign agents and domestic opportunists became one of the major subplots of Donald Trump’s victory and sparked a series of high-profile congressional inquiries. Despite all of the backlash, all the scrutiny, all the promises made by the likes of Facebook CEO Mark Zuckerberg and Twitter CEO Jack Dorsey to do better, the boogeymen that reared their head then are still snarling today. That’s dispiriting, because the tech companies had two years to prepare, and untold resources at their disposal. Facebook even had a well-staffed election “war room” tasked with finding and addressing the very kinds of hoaxes that continued to crop up throughout the election cycle. If they haven’t fixed things by now, well: When will they? The answer is probably “never.”…”
“At first grimace, the role of social media in the 2018 U.S. midterm elections looked a lot like the role it played in the 2016, when the hijacking of tech platforms by foreign agents and domestic opportunists became one of the major subplots of Donald Trump’s victory and sparked a series of high-profile congressional inquiries. Despite all of the backlash, all the scrutiny, all the promises made by the likes of Facebook CEO Mark Zuckerberg and Twitter CEO Jack Dorsey to do better, the boogeymen that reared their head then are still snarling today. That’s dispiriting, because the tech companies had two years to prepare, and untold resources at their disposal. Facebook even had a well-staffed election “war room” tasked with finding and addressing the very kinds of hoaxes that continued to crop up throughout the election cycle. If they haven’t fixed things by now, well: When will they? The answer is probably “never.”…”
The New York Times – Machine learning algorithms don’t yet understand things the way humans do — with sometimes disastrous consequences. “…As someone who has worked in A.I. for decades, I’ve witnessed the failure of similar predictions of imminent human-level A.I., and I’m certain these latest forecasts will fall short as well. The challenge of creating humanlike intelligence in machines remains greatly underestimated. Today’s A.I. systems sorely lack the essence of human intelligence: understanding the situations we experience, being able to grasp their meaning. The mathematician and philosopher Gian-Carlo Rota famously asked, “I wonder whether or when A.I. will ever crash the barrier of meaning.” To me, this is still the most important question. The lack of humanlike understanding in machines is underscored by recent cracks that have appeared in the foundations of modern A.I. While today’s programs are much more impressive than the systems we had 20 or 30 years ago, a series of research studies have shown that deep-learning systems can be unreliable in decidedly unhumanlike ways. I’ll give a few examples.
The bareheaded man needed a hat” is transcribed by my phone’s speech-recognition program as “The bear headed man needed a hat.” Google Translate renders “I put the pig in the pen” into French as “Je mets le cochon dans le stylo” (mistranslating “pen” in the sense of a writing instrument). Programs that “read” documents and answer questions about them can easily be fooled into giving wrong answers when short, irrelevant snippets of text are appended to the document. Similarly, programs that recognize faces and objects, lauded as a major triumph of deep learning, can fail dramatically when their input is modified even in modest ways by certain types of lighting, image filtering and other alterations that do not affect humans’ recognition abilities in the slightest…”